Source: Freepik
Organisations need reliable and easily accessible data to keep information flowing, make business decisions, and innovate. Privacy concerns, however, are increasing in line with the need for innovation and data utilisation.
Data utilisation is a key concept for AI projects, as they require large amounts of data to be trained and tested. The demand for AI is booming in almost every sector, especially healthcare, finance, manufacturing, and robotics. However, EU GDPR (General Data Protection Regulation) and other regulations strictly restrict the use and sharing of sensitive data such as customer names, addresses, bank account numbers, and health records. Currently, Europe, the UK, and North America have adapted advanced data privacy regulations, and other countries are stepping up to take similar actions as well. However, these regulations are challenging for organisations and hamper their data-driven product development. Synthetic data is becoming the most promising solution to overcome these restrictions.
Defining the Synthetic Data
Synthetic data is the artificially generated data by computer algorithms or simulations that carries the statistical properties and structure of the original data. Compared to conventional anonymization techniques, synthetic data does not preserve a direct relationship with the original data and ensures optimum privacy and utilisation, and allows scalability.
Several conventional data anonymization techniques are being used to comply with privacy regulations. Data generalisation aims at anonymization by excluding some parts of the data. An example of this technique would be removing the day from the date of birth while keeping the month and year. In data masking, privacy is provided by replacing a character in the dataset with different variables such as “x” or “#”. Data shuffling, also called data swapping or permutation, rearranges the dataset, so it does not overlap with the original dataset while preserving the logical relationships. In pseudonymization, the identity of the data subject is changed; John Smith becomes David Brown. Anonymization techniques can be evaluated and classified in different ways based on how sensitive the datasets are, their use, and how much accuracy is expected. Dr. Fintan Nagle, an ML scientist and one of co-founders of Hazy, and Sofiane Mahiou, Head of Data Science at Hazy, have published Anonymisation Literature Review in order to create common literature on anonymization concepts and explain the main algorithms.
Although there are many alternatives to anonymizing data, this process is still time-consuming and does not fully meet the requirements as it can be reverse engineered and re-identified. In addition, while the data is being anonymized, the information it contains is destroyed and the quality of data decreases. This directly lowers the performance and reliability of AI/ML models.
Advantages of Synthetic Data
Synthetic data, generated by computer algorithms trained on real-world data, stands out as the most versatile solution among privacy-enhancing technologies, given its the following advantages:
- Privacy: Synthetic data relies on creating data from scratch rather than modifying the existing datasets. It minimises the risk of re-identification.
- Quality: Real-world data is prone to errors as it requires manual labelling and biases, as it contains unbalanced datasets. Synthetic data automatically balances outliers and creates bias-free datasets, enabling more reliable inferences.
- Reduced time and cost: Synthetic data eliminates the long and costly burden of accessing data by optimising the processes of data collection, preparation, and labelling.
- Scalability: Synthetic data provides a scalable approach by expanding datasets when data is scarce. It also allows existing small datasets that are currently ignored to be augmented and used.
- Monetisation: Organizations can monetise their synthetic data through the marketplace and enrich their datasets by combining them with third-party data.
- Simplicity: Synthetic data tools do not require the end user to have high data science skills. They automatically update inputs in the background and continue synthetic data generation for required cases.
Categorisation of Synthetic Data
Synthetic data can be divided into three categories according to their convergence with real data:
- Fully synthetic data: Data that is fully synthetic and does not contain any information from real data. It is easier to control, giving the user more manoeuvrability and a high level of privacy protection.
- Partially synthetic data: Data is created by replacing selected parts of real data with synthetic data and rearranging the rest.
- Hybrid synthetic data: Data combining real and synthetic data. It provides the advantages of both types but takes longer to process and requires more memory.
Synthetic data can also be classified according to the format of the data. The market map from Elise Devaux divides synthetic data providers into two groups: structured and unstructured data providers. Structured data refers to tabular data such as names, addresses, or credit card numbers that are arranged in rows and columns. In contrast, unstructured data refers to textual data such as audio and media files that are not configured with a predefined format.
Synthetic Data Generation Techniques
There are three main techniques to generate synthetic data:
- Drawing numbers from a distribution: This approach relies on data scientists observing the statistical distribution of real-world data and reproducing fake data using a statistical probability distribution method. However, it should be noted that the model's accuracy is directly proportional to the expertise of the data scientists.
- Agent-based modelling: Agent-based model is a simulation modelling technique that consists of autonomous decision-making entities called agents. Each agent observes and understands the system and then interacts with each other to generate data from the same model.
- Generative models: Generative models are deep learning models that can work with larger and more complex datasets to create highly correlated and indistinguishable datasets. The most common approach in generative models is Generative Adversarial Networks (GANs). GANs consist of two sub-models, a generator, and a discriminator, that works and evolves against each other. The generator is responsible for generating synthetic data. Discriminator, on the other hand, is trained on real data and is responsible for identifying the distinction between synthetic data and real data. When the discriminator detects fake data, it notifies the generator to modify its modeling. The generator continues the process until the discriminator cannot distinguish between the two datasets. The figure below shows the process of creating a synthetic image of money with the GAN architecture.
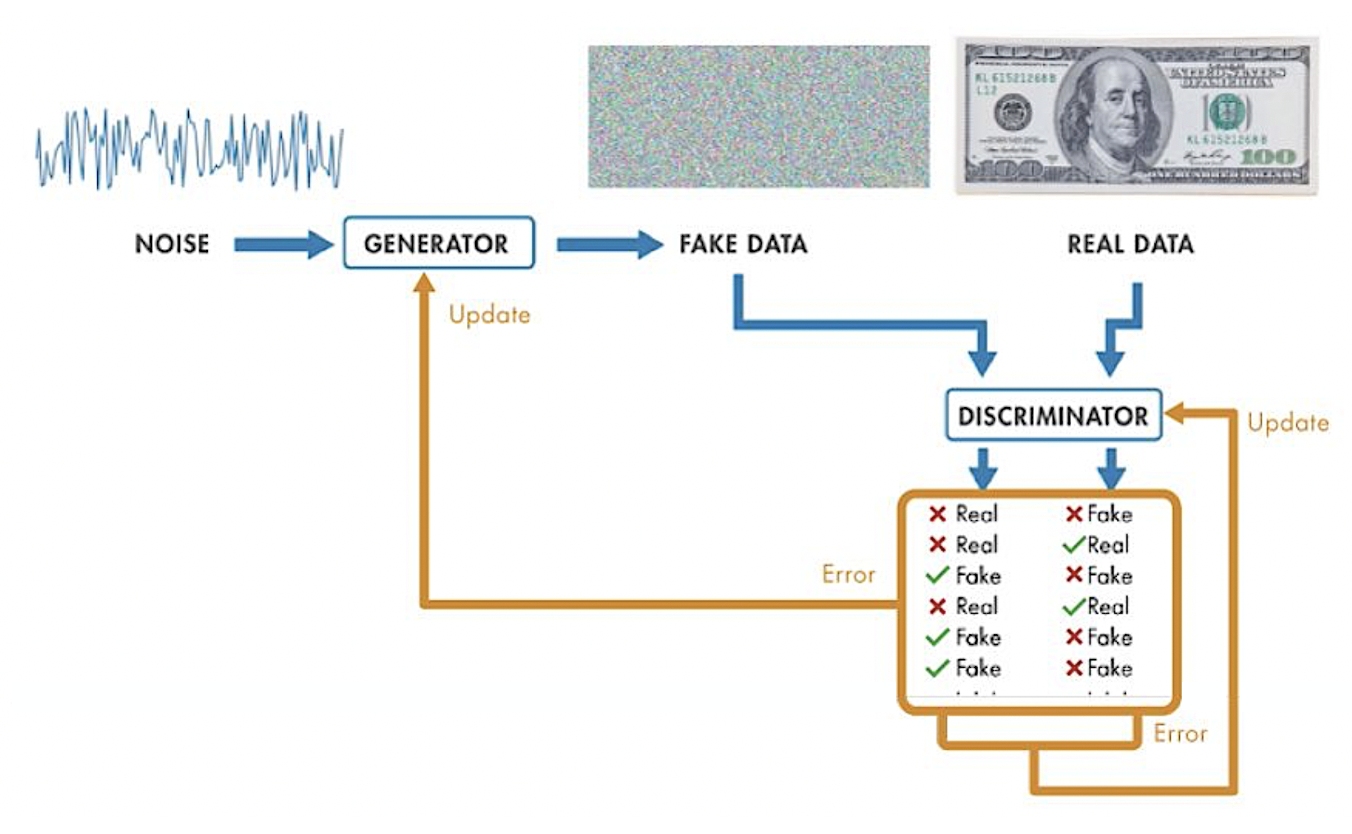
Data synthesis process using GANs. Source:Mathworks
The Key Uses of Synthetic Data
Synthetic data providers can generate the required data within hours and make it ready for use. The following real world examples (please follow their links for the details in each use case) are among the most common use cases of synthetic data;
- Testing: A software or product can be tested before it is deployed in the real world. A synthetic data provider, Mostly AI works with a European bank to develop its mobile banking app. The bank creates synthetic customer profiles using the algorithm of Mostly AI and improves the user experience.
- Training AI and ML models: Simulations with different parameters can be created to train AI and ML models efficiently. Google’s Waymo uses synthetic data to train its ML models for its self-driving vehicles.
- Data Sharing: Synthetic data can be freely shared within an organisation without compliance concerns, facilitating collaboration between teams and speeding up internal processes. Synthetic data also removes restrictions on external data sharing, allowing innovation teams to evaluate new technologies and stay ahead of the competition. A bank, Nationwide, partners with Hazy, a synthetic data provider, and drastically reduces the time required to provide data to third parties.
Industry-specific use case examples:
- Banking and financial services: The use cases of synthetic data in financial institutions include fraud modelling, financial crime, AML, credit risk, operational risk, and asset management. Datasets encountered in fraud modelling, and financial crime are highly imbalanced, as the number of transactions labelled as fraud takes up very little space, with less than 1% of all datasets. Synthetic data can generate balanced datasets by increasing the number of "fraud" transactions and ensuring accurate modelling. American Express uses synthetic data to overcome fraudulent behaviours.
- Healthcare and pharmaceuticals: Strict privacy regulations, difficulty accessing patient data, and data scarcity are some obstacles that must be overcome for the healthcare industry to thrive. Synthetic data can be trained on real patient data and allow researchers to work on clinical trials where real patient data is not yet available, or there is insufficient data to reach meaningful conclusions. The use of synthetic data in healthcare contributes to a more accurate and personalised healthcare approach, enables rare diseases to be predicted much earlier, and accelerates drug discovery. DNA sequencing company, Illumina partners with Gretel.ai, a synthetic data provider, to create complex datasets for use in genomic research.
- Automotive: Autonomous vehicle companies do not have the resources, vehicles, and time to test every scenario that their vehicles may encounter in the real world. Synthetic data can mimic the real world with thousands of different simulations to avoid collisions and enable the development of AVs. Autonomous vehicle startup Waabi uses synthetic data to stress test Waabi Drivers and give them driving ability.
The Market’s State
Synthetic data fuels critical industries with large volumes of highly sensitive data, such as Finance, Healthcare, Manufacturing, Automotive and Robotics, Security, HR, Marketing, and Insurance. Recent research by Cognilytica has shown that the Synthetic Data market continues to expand with 76 vendors in the landscape and is expected to reach $1.15 billion by the end of 2027, from $110 million in 2021.
Synthetic data startups have received a significant amount of investment in recent years. Datagen closed a $50 million Series B round led by Scale Venture Partners, Gretel.ai raised a $50M Series B round led by Anthos Capital, Mostly AI received $25M in Series B round led by Molten Ventures, Hazy raised a $3.5M Seed+ round led by Notion Capital, and Synthesized secured $2.8m in Seed funding led by IQ Capital and Mundi Ventures.
As startups continue to emerge in the market, big players such as Google, AWS, Facebook, and NVIDIA are also positioning themselves in the market by investing heavily in synthetic data. In 2021, Facebook acquired New York-based synthetic data startup AI.Reverie to deal with privacy issues and improve its computer vision programs. The same year, BMW created a virtual factory facility using NVIDIA's synthetic data generation engine, the NVIDIA Omniverse. Before building the factory in the real world, BMW’s team could understand and improve how assembly workers and robots work together to efficiently build cars with simulations created on the platform.
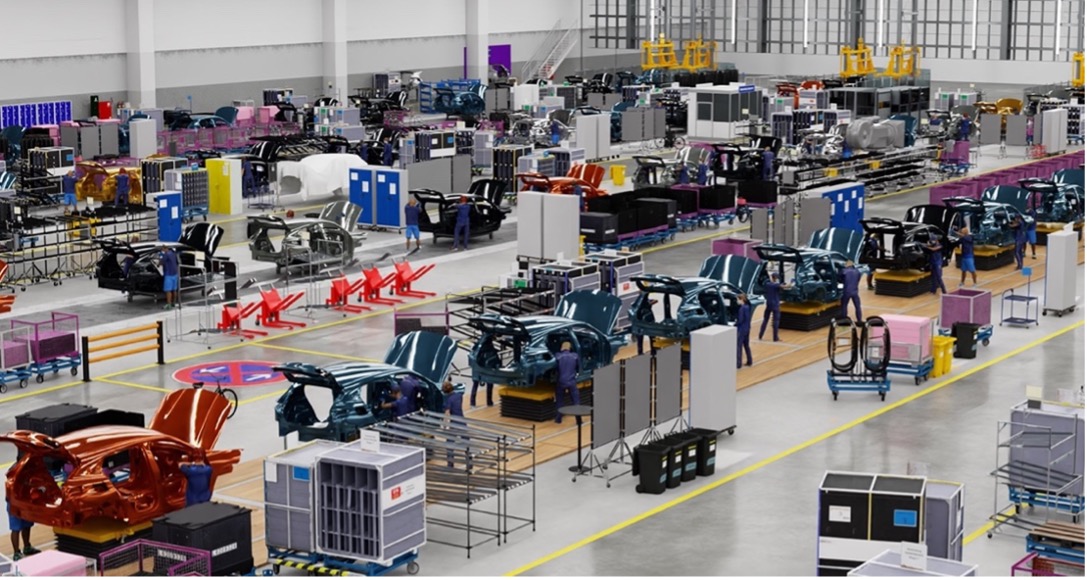
A digital twin of BMW’s assembly system powered by Omniverse. Source: NVIDIA
Last month, BBVA AI Factory took a similar step and started working with Spanish startup Dedomena to address privacy challenges and improve the development of AI. The latest development in the market is that SageMaker Ground Truth, AWS' data labelling service, now supports synthetic data generation, as AWS announced last week.
Future Prospects
Synthetic data plays a crucial role in revolutionising AI and ML model training. The momentum is real in its adoption. It is needed in every field where data is used. The sooner organisations adopt synthetic data and create their trained dataset basis, the better because tightening regulations and the unique advantages of synthetic data will inevitably make synthetic data a necessity rather than an option for any company, regardless of industry, size, or use case.
References
- Devaux, E. (2022, March 29). Types of synthetic data and 4 real-life examples (2022). Statice. https://www.statice.ai/post/types-synthetic-data-examples-real-life-examples
- Dilmegani, C. (2022, June 14).Synthetic Data Generation: Techniques, Best Practices & Tools. AIMultiple. https://research.aimultiple.com/synthetic-data-generation/
- Nagle, F. (2018, March 1). Anonymisation Literature Review. Hazy. https://hazy.com/blog/2018/03/01/anonymisation-literature-review/
- Singh, K. (2021, May 12). Synthetic Data — key benefits, types, generation methods, and challenges. Medium. https://towardsdatascience.com/synthetic-data-key-benefits-types-generation-methods-and-challenges-11b0ad304b55
- Synthetic Data for Machine Learning: its Nature, Types, and Ways of Generation. (2022, March 22). AltexSoft. https://www.altexsoft.com/blog/synthetic-data-generation/
- Synthetic Data Generation Techniques. (n.d.). Turing. https://www.turing.com/kb/synthetic-data-generation-techniques
- Synthetic Data: The Complete Guide. (n.d.). Datagen. https://datagen.tech/guides/synthetic-data/synthetic-data/
- Synthetic Data vs Other Privacy Preserving Technologies. (n.d.). Datomize. https://www.datomize.com/resources/synthetic-data-vs-other-privacy-preserving-technologies/
- Vieira, A. (2020, August 11).The Beauty of GANs: Sharing insights without sharing data. Hazy. https://hazy.com/blog/2020/08/11/the-beauty-of-gans/
- Watson, A. (2022, March 24). How to Generate Synthetic Data: Tools and Techniques to Create Interchangeable Datasets. Gretel. https://gretel.ai/blog/how-to-generate-synthetic-data-tools-and-techniques-to-create-interchangeable-datasets