Pictured from left: Dr. Luke Robinson, Head of Science and Co-Founder, Sofiane Mahiou, Head of Data Science, Harry Keen, CEO and Co-Founder, Andrew Keen, COO, Carl Tishler, CPO
As the leading synthetic data platform utilizing deep learning to replicate the systematic properties of real-world data, Hazy is an obvious match for ACT. We are happy to be a part of Hazy’s USD 9 million Series A round led by Conviction VC and joined by existing investors including UCL Technology Fund, M12 (Microsoft), Wells Fargo, and Nationwide Building Society. We look forward to partnering with the team in their mission to bring the true value of synthetic data to light.
The Dilemma: Privacy-Utility Tradeoff
In the ever-evolving technology landscape, organizations must access and utilize data in order to innovate and remain competitive. Internal data sharing and usage are especially critical for product development and training of AI/ML models. However, the direct use of data containing personally identifiable information (PII) such as usernames, credit card information, or passwords in any operation within or outside the organization is limited by the European Union’s General Data Protection Regulation (GDPR), the Artificial Intelligence Act, and other similar regulations. The GDPR Fines Tracker released by Privacy Affairs shows that the GDPR fines issued by European data protection authorities have increased from a total of EUR 158.5 million in 2020 to EUR 2.3 billion as of January 2023. Thus, while the ability of organizations to generate value through data analysis has been growing exponentially, the use and sharing of data are associated with substantial regulations-compliance challenges and costs. Data privacy-enhancement methods, such as masking, generalization, and shuffling that have been employed to anonymize sensitive data are often rendered useless due to the risk of user re-identification and/or the unsatisfactory preservation of the original data’s statistical properties. Thus, synthetic data has emerged as a viable solution able to address the older methods’ drawbacks. The reliable and high-quality datasets enable organizations to comply with regulations and pave the way for innovation and value creation.
In the ever-evolving technology landscape, organizations must access and utilize data in order to innovate and remain competitive. Internal data sharing and usage are especially critical for product development and training of AI/ML models. However, the direct use of data containing personally identifiable information (PII) such as usernames, credit card information, or passwords in any operation within or outside the organization is limited by the European Union’s General Data Protection Regulation (GDPR), the Artificial Intelligence Act, and other similar regulations. The GDPR Fines Tracker released by Privacy Affairs shows that the GDPR fines issued by European data protection authorities have increased from a total of EUR 158.5 million in 2020 to EUR 2.3 billion as of January 2023. Thus, while the ability of organizations to generate value through data analysis has been growing exponentially, the use and sharing of data are associated with substantial regulations-compliance challenges and costs. Data privacy-enhancement methods, such as masking, generalization, and shuffling that have been employed to anonymize sensitive data are often rendered useless due to the risk of user re-identification and/or the unsatisfactory preservation of the original data’s statistical properties. Thus, synthetic data has emerged as a viable solution able to address the older methods’ drawbacks. The reliable and high-quality datasets enable organizations to comply with regulations and pave the way for innovation and value creation.
The Way Out: Synthetic Data
Synthetic data is algorithmically generated data that does not contain any real information while preserving to a high degree the statistical properties, patterns, and correlations present in the original data.
Synthetic data is algorithmically generated data that does not contain any real information while preserving to a high degree the statistical properties, patterns, and correlations present in the original data.
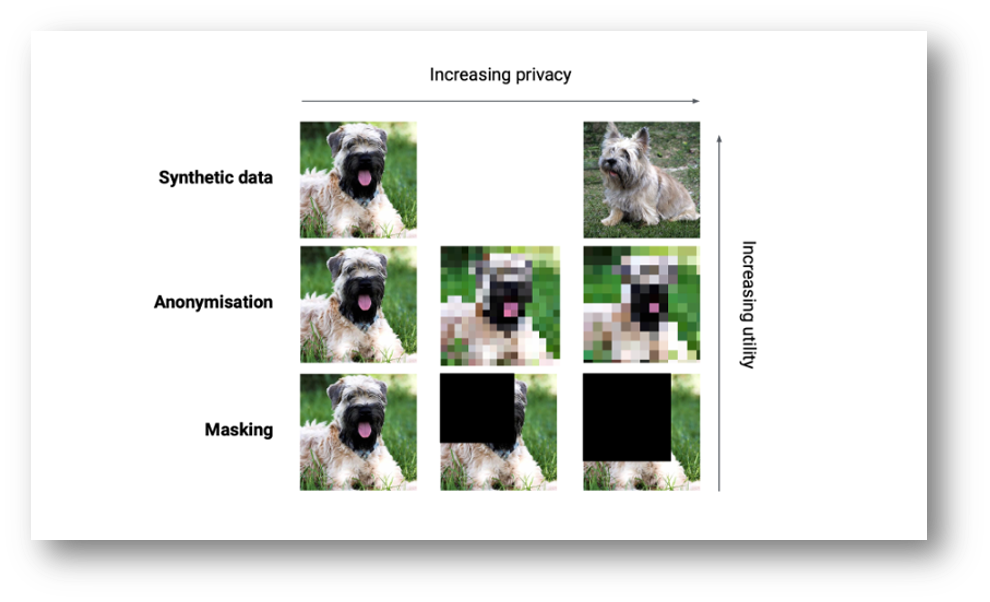
Synthetic data does not preserve a direct relationship with the original data and ensures optimum privacy and utilization. Source: Hazy
Research published by Cognilytica states that the synthetic data market exceeded USD 110 million in 2021 and is expected to reach USD 1.15 billion by the end of 2027. The market is driven by tightening regulations and organizations' desire for innovation. It is recognized that the initial demand for synthetic data is generated by certain industries where massive amounts of sensitive data exist, such as financial services, telecommunications, public administration, defense, retail, and market research.
Despite the rapidly growing market and wide range of use cases, the absolute challenge is to generate real results with synthetic data. This is where Hazy comes in.
Despite the rapidly growing market and wide range of use cases, the absolute challenge is to generate real results with synthetic data. This is where Hazy comes in.
Hazy: Redefining Synthetic Data
Founded in 2017 as a University College London AI spinout, Hazy aims to help organizations reduce compliance risk and accelerate data innovation by making high-quality synthetic data available. Hazy offers an integrated workflow tool that automatically anonymizes and periodically updates datasets to simplify the management of data privacy and eliminate the reliance on manpower in the process.
Hazy's software platform creates a digital twin of the original dataset containing the latter’s defining statistical properties. The new dataset can be used in various applications, such as data migration, portability, testing, and analytics. What differentiates Hazy from its competitors is its proven success in working on complex multi-table datasets and generating sequential and time-series synthetic data. Its unique generator-based architecture allows users to train a synthetic data generator on-site anywhere, by transporting the ML model within the organization, or even externally. Hazy's technology has demonstrated its value by enabling the testing of 100x new vendors each year and provisioning data 20x faster in different use cases for customers in the financial and automotive industries respectively.
Founded in 2017 as a University College London AI spinout, Hazy aims to help organizations reduce compliance risk and accelerate data innovation by making high-quality synthetic data available. Hazy offers an integrated workflow tool that automatically anonymizes and periodically updates datasets to simplify the management of data privacy and eliminate the reliance on manpower in the process.
Hazy's software platform creates a digital twin of the original dataset containing the latter’s defining statistical properties. The new dataset can be used in various applications, such as data migration, portability, testing, and analytics. What differentiates Hazy from its competitors is its proven success in working on complex multi-table datasets and generating sequential and time-series synthetic data. Its unique generator-based architecture allows users to train a synthetic data generator on-site anywhere, by transporting the ML model within the organization, or even externally. Hazy's technology has demonstrated its value by enabling the testing of 100x new vendors each year and provisioning data 20x faster in different use cases for customers in the financial and automotive industries respectively.
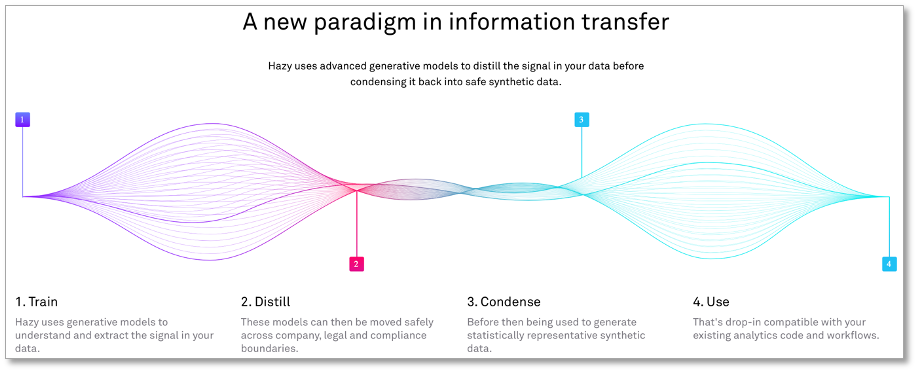
Source: Hazy
Besides demonstrating high performance in complex data structures, Hazy offers several additional enhancements, such as rare event prediction and dataset balancing. Hazy's algorithms allow the ML model to uniquely transfer its learned knowledge between similar events to obtain meaningful results from events that have not yet occurred or for which the user has very limited data. Finally, Hazy’s platform provides the opportunity for customers to generate an additional revenue source by monetizing their synthetic data. With the Hazy synthetic data marketplace, users will be able to combine, sell, and exchange first and third-party data.
Hazy is working with some of the most notable players in the finance, telecom, and market research industries, including Nationwide Building Society, Vodafone, and Accenture, to name a few. Behind Hazy, there is a visionary team led by co-Founders Harry Keen and Luke Robinson, eager to take the company to the next level in synthetic data. Hazy has proven its technology by winning multiple competitions and awards, including Microsoft’s Innovate.AI, and has solidified its reputation by being recognized by the well-respected organizations Gartner and CB Insights.
The Road Ahead
Hazy plans to use the proceeds of the current investment round to scale its sales and marketing, grow in different verticals, as well as expand its team. As ACT Venture Partners, we look forward to joining the team's ambitious journey to pushing the frontier of synthetic data.
The Road Ahead
Hazy plans to use the proceeds of the current investment round to scale its sales and marketing, grow in different verticals, as well as expand its team. As ACT Venture Partners, we look forward to joining the team's ambitious journey to pushing the frontier of synthetic data.
References
- Arthur, J. (2019, May 1). Anonymised Data. https://hazy.com/blog/2019/05/01/anonymised-data/
- Haerens, E. (2022, May 19). GDPR in a post-Covid landscape. https://hazy.com/blog/2022/05/19/gdpr-in-a-post-covid-landscape/
- Mikhalev, A. (2020, December 16). Nationwide unlocks rapid innovation with synthetic data. https://hazy.com/blog/2020/12/16/nationwide-building-society-case-study/
- Riggins, J., & Keen, H. (n.d.). Hazy | Shake’Up — How synthetic data could have let us prepare for this pandemic? RiskInsight. https://www.riskinsight-wavestone.com/en/2020/07/hazy-shakeup-how-synthetic-data-could-have-let-us-prepare-for-this-pandemic/
- Vieira, A. (2020, April 27). Generating synthetic data with referential integrity using GANs. https://hazy.com/blog/2020/04/27/generating-synthetic-data-with-referential-integrity-using-gans/